Emerging Architectures in AI
Liquid AI, Inference, Memory, Application layers updates, semiconductor potential. Super Datacenters.
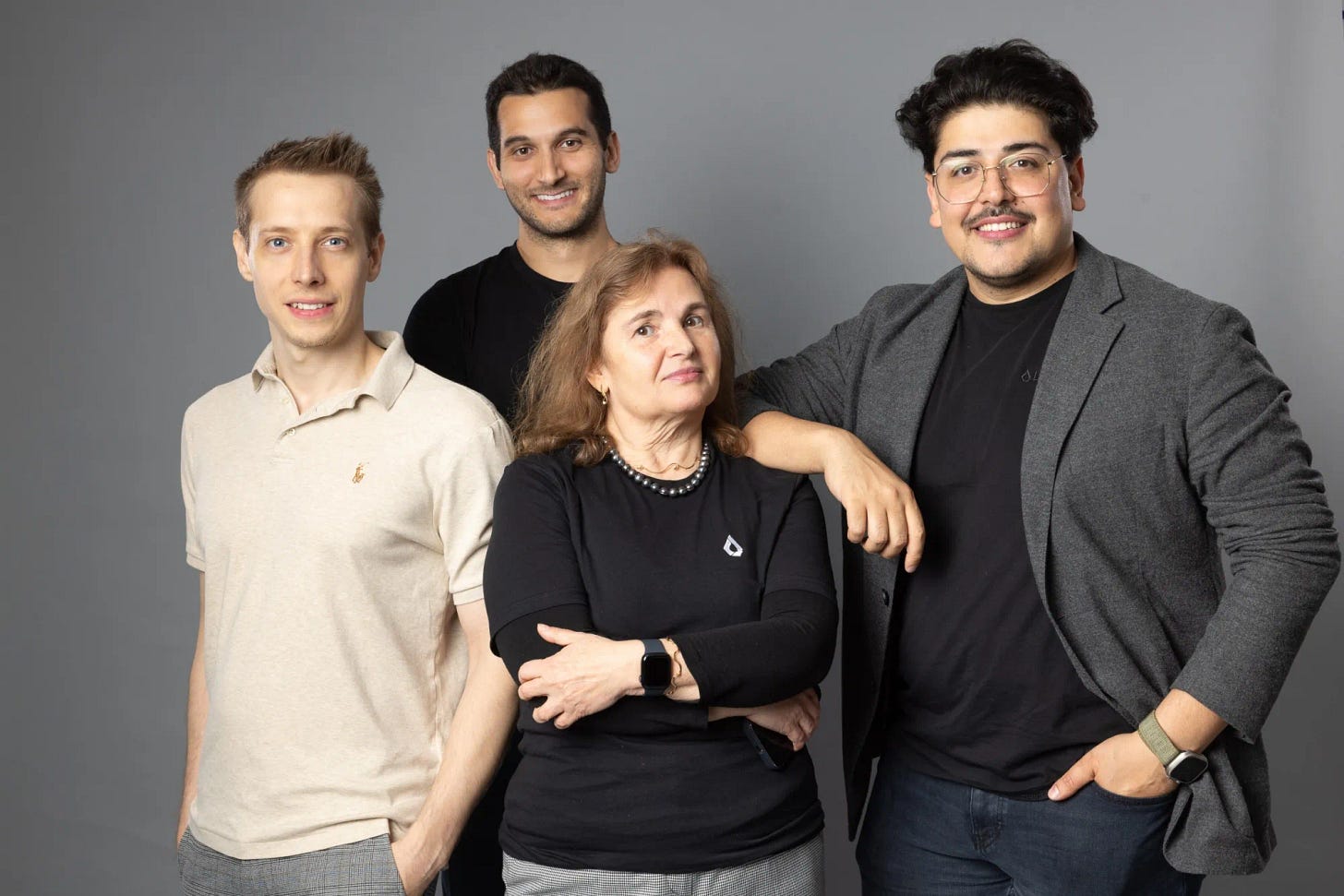
AI Supremacy is a reader supported publication, sharing our work helps us grow and is very much appreciated.
There’s something to be said for finding emergent architectures or new ways of doing things. Take Groq’s Language Processing Unit (LPU) a specialized AI accelerator designed to optimize the performance of artificial intelligence workloads, particularly in inference tasks. Or Sandbox AQ’s Large Quantitative Models, LQMs.
Or take Sakana AI’s Neural Attention Memory Models (NAMMs) that they say are a new kind of neural memory system for Transformers that not only boost their performance and efficiency but are also transferable to other foundation models, without any additional training.
When we think of what’s ahead, Ilya Sutskever gave us a nice summary recently at NeurIPS, giving us additional context of the recent history of AI. If he confirmed that LLMs scaling has plateaued in terms of training data, this bottleneck allows for other innovations to take place. Deep learning never rests, even in pushing the boundaries of things like drug development and the future of biotechnology.
Broadcom believes hyperscalers will deploy 1,000,000 XPU clusters across a single fabric as soon as 2027, which is 10x that of which xAI has today in terms of datacenter AI compute. In just a few short years, Scaling laws with be powered by a greater focus on inference, memory, Agentic AI, and on the application layer with tremendously more capable GPUs and AI infrastructure. Broadcom itself is working with the likes of ByteDance, Apple and OpenAI on custom AI chips.
In the decade ahead we are somewhat likely to find new kinds of emergent architectures that expand what we are able to do with LLMs or perhaps will be more efficient and open up new avenues, even as small language models, and world models continue to expand our horizons.
AI pioneer Fei-Fei Li’s World Labs has raised $230 million to build “large world models” (LWMs) with a lot of experimentation at places like Google on world simulators. Google recently announced Genie 2, a foundation world model capable of generating an endless variety of action-controllable, playable 3D environments for training and evaluating embodied agents.
Heading into 2025 we don’t know exactly what to expect. We do know that capex is only going to increase and national competition could accelerate innovation. According to one estimate, the global AI market reached $196.63 billion in 2023 and could be worth $1.81 trillion by 2030. In Short, the AI market is expected to grow at a CAGR of 36.6% from 2024 to 2030 reaching the remarkable figure of 1,811.75 million USD.
Recently Omar Sanseviero of Hugging Face has released a book worth checking out if you dabble with LLMs, called Hands-On Generative AI with Transformers and Diffusion Models. It’s available at O’Reilly and Amazon. I’m not an affiliate, only an enthusiast. There are so many good books coming out around LLMs, those by
in my view are great.I want to talk especially about AMD’s best on Liquid AI and Ayar Labs and what their architectures might mean for the future.
Upgrades at the Application Layer
Keep reading with a 7-day free trial
Subscribe to AI Supremacy to keep reading this post and get 7 days of free access to the full post archives.