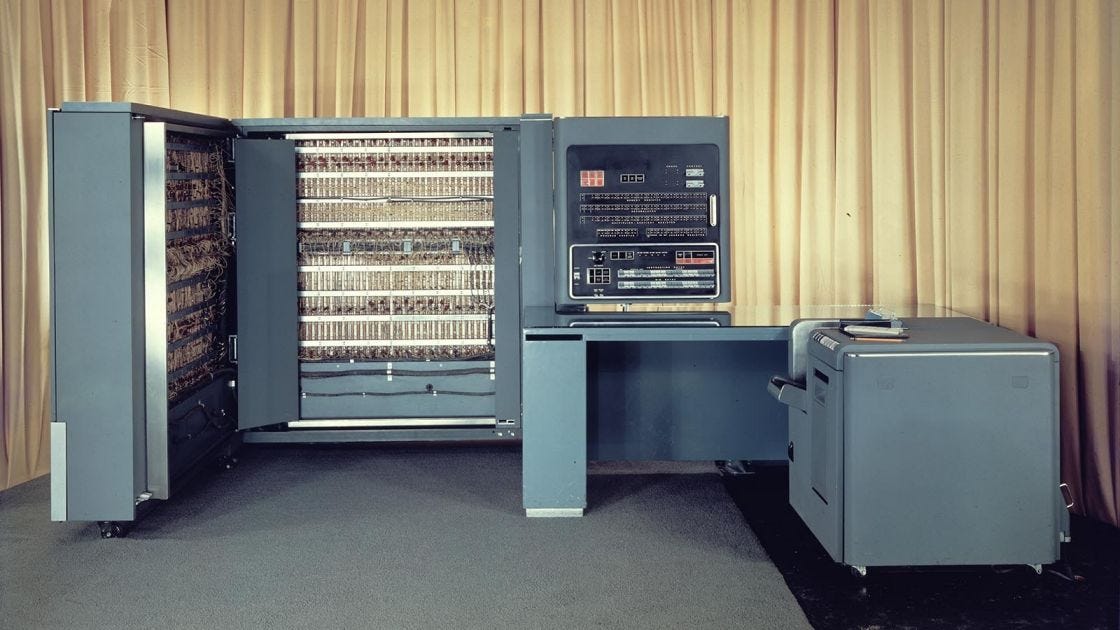
History is a funny thing, on some days the goalposts are moved.
Papers on AGI of Note | Related 📑
Sparks of AGI (2023)
Evaluation of OpenAI o1: Opportunities and Challenges of AGI (2024)
Managing extreme AI risks amid rapid progress (2024)
An Approach to Technical AGI Safety and Security (2025)
I consider
my lead AGI reporter, if such a thing could exist. It’s important we keep debating AGI and ASI because it has ramifications on the global economy and society as a whole. If one day, AI were to become sentient, self-motivated and awaken from the ghost in the machine what would it attempt to do?The Rise of Autonomous Systems
The main commercial AI company of our times, “OpenAI’s mission is to ensure that artificial general intelligence (AGI)—by which we mean highly autonomous systems that outperform humans at most economically valuable work—benefits all of humanity.” - OpenAI Charter
Mimicking the Human Brain in Machine Intelligence
As of 2025, the current definition of AGI is approximate: “AGI, or Artificial General Intelligence, refers to a hypothetical type of AI that possesses human-level intelligence, capable of understanding, learning, and applying knowledge across various domains, just as a human can.
Unlike narrow AI, which is designed for specific tasks, AGI aims to simulate the cognitive abilities of the human brain, including reasoning, problem-solving, and adapting to new situations.” This isn’t a perfect definition, but it will have to do for today’s exploration.
Summary: AGI is a hypothetical type of AI that possess human-level intelligence:
Can Generalize | ⚖️
Has Common Sense | 🤷
Is Adaptable | 🧩
Can Problem Solve | ✨
Can self-learn/self-teach | 🚦
Has human level cognitive abilities | 👩🏻
Bio: Harry Law writes Learning From Examples, a history newsletter about the future. AI is a major focus, but he writes about any episode that helps us think more clearly about technological change. He’s a PhD candidate at the University of Cambridge and a former researcher at Google DeepMind.
Examples of recent articles by Harry, titles:
His “Learning From Examples”, a Newsletter on Substack for more of his refreshing essays on AI and the history of technology. What follows is his essay of May, 2025. By Harry Law, who is spending his time reading and writing his way through a PhD at the Department of History and Philosophy of Science at the University of Cambridge.
Levels of AGI according to 2023
By
, May, 2025AGI is mainstream now
In 1964, U.S. Supreme Court Justice Potter Stewart famously delivered one of the most enduring phrases in American legal history. Faced with the task of defining hard-core pornography, he shrugged off abstraction and wrote simply: ‘I know it when I see it.’
Some things resist precise definitions. In the world of artificial intelligence, that thing is Artificial General Intelligence (AGI). The problem is that AGI is basically in the eye of the beholder. When I worked at Google DeepMind, I generally thought about it according to the ‘levels of AGI’ framework that uses the proportion of skilled adults that a model can outperform as a baseline. Doing so gives us several different types of AGI (e.g. expert, virtuoso etc.) rather than a binary definition where we’re forced to ask: ‘so, AGI, yes or no?’
This essay isn’t about definitions. In fact, I generally lament that pretty much every conversation about AGI has the unfortunate tendency to converge on questions about what the technology is or isn’t. Perhaps there should be a law for this, like Godwin’s law but for ultra-powerful AI systems.
I’m more interested in how the term, unstable as it might be, migrated from the fringes of respectability to something bordering on the normal. We are, after all, talking about the big one, the point of no return, one technology to rule them all.
When I first started working in AI in 2018, basically no-one talked about AGI. Yes, if you were online enough, you knew that certain internet subcultures were interested in it – but it is not as if the term was gobbling up column inches like it does today.
Then came some movement. Google DeepMind (then DeepMind) was probably the first lab that took AGI seriously in the early 2010s, followed by OpenAI during the middle of the decade. But the genesis of AGI goes back further back than that.
The earliest meaningful example of the term I’m aware of was by Mark Gubrud, who used it in a 1997 discussion of the implications of fully automated military production and operations. Otherwise, I’ll defer to Ben Goertzel’s blog on the subject:
‘In 2002 or so, Cassio Pennachin and I were editing a book on approaches to powerful AI, with broad capabilities at the human level and beyond, and we were struggling for a title. The provisional title was “Real AI” but I knew that was too controversial. So I emailed a bunch of friends asking for better suggestions. Shane Legg, an AI researcher who had worked for me previously, came up with Artificial General Intelligence. I didn’t love it tremendously but I fairly soon came to the conclusion it was better than any of the alternative suggestions. So Cassio and I used the term for the book title (the book “Artificial General Intelligence” was eventually published by Springer in 2005), and I began using it more broadly.’
It sounds almost quaint for a subject that eats up so much of the AI discourse today. After all, OpenAI famously included AGI in its founding charter in 2015 and has–on and off–been using the term with increasing regularity since. Earlier this year, the company said it is ‘confident we know how to build AGI as we have traditionally understood it’ and that 2025 is the year that AI agents will ‘materially change the output of companies’.
Those two things aren’t the same, but the broader point is that OpenAI thinks that AGI is coming sooner rather than later. Mustafa Suleyman of Microsoft reckons that AGI is ‘more than two years away’, DeepMind’s Demis Hassabis says ‘in the next five to ten years’, and Anthropic's Dario Amodei says 2026 or 2027 is the moment that we’ll ‘get there’.
Away from the big labs, start-ups are also getting in on the action. LLM sceptic François Chollet launched a new venture, Ndea, in January 2025 whose mission is to ‘develop and operationalize AGI’. Ex-OpenAI CTO Mira Murati founded Thinking Machines Lab in February following her departure from the company, which argues that scaling to the ‘highest level’ of capability is the goal (they don’t namedrop AGI specifically).
Sticking with the ex-founding team of OpenAI, former chief scientist Ilya Sutskever co-founded Safe Superintelligence (SSI) whose mission is to leapfrog AGI and head straight for superintelligence.
We should take all of these claims seriously. While AGI itself might be, as Dario Amodei says, something of a marketing term – that doesn’t mean that we shouldn’t view reflections on the development of highly capable AI systems as significant stuff. Call it AGI or call it something else, the people building the systems think that something powerful is just around the corner.
They have good reason to think that. Scaling (that is, simply making the systems we have right now bigger) might not deliver the goods alone, but a few important developments will likely make up for that.
The first is multimodality. I remember playing with DALLE-2 when it came out and thinking it was hugely impressive. Now it looks horribly dated. The same goes for the versions of image generator Midjourney, whose outputs have gone from ‘uncanny horrors’ to ‘more or less photorealistic’ in the space of two years. Never mind video, audio, and coding. The last one is the reason the labs are so confident, because sharp coding abilities could dramatically speed up the development of models. And no, before you worry that the scripts aren’t all that, the quality of code doesn't need to be perfect – it just needs to be good enough.
Another reason is test-time compute. For reasons unclear, it turns out that a model can talk itself through generating an output to dramatically increase performance on certain tasks. This isn’t great on every occasion–in fact, reasoning models can be deeply annoying on writing jobs or in conversation–but it is enough to boost performance on some of the trickiest benchmarks around. Yes, benchmarks aren’t everything, but they do matter.
Finally, there’s the structure of the systems themselves as distinct from the underlying model. My own view, taking a leaf out of Marvin Minsky’s book, is that the first AGIs will be constellations of smaller models and tools. One for image generation. One for reasoning. One for search. And so on. This takes the pressure off a single model being all things to all people, and allows it to take up a role as the conductor within a broader collection of useful devices. This is basically what agents do, which I wrote about in my last article for AI Supremacy (e.g. Manus AI).
There’s also a self fulfilling prophecy here. AGI, for all its fuzziness, has become a magnet for capital, talent, and attention. The result is a kind of recursive acceleration: the more confidently labs talk about AGI, the more seriously investors take the prospect, the more money flows in, and the more new products vindicate that confidence.
Timelines are collapsing 🕒
For these reasons, timelines are getting shorter. Metaculus currently has the average prediction for the arrival of AGI within the decade. The labs, as we saw earlier, think it’s likely to come much sooner than that. If you don’t believe that AGI is anything other than a cynical marketing ploy then I doubt much will convince you, but it is curious to think about some of the more recent statements on safety from the labs in this context.
Anthropic, for example, thinks that we’re looking at ‘a race between interpretability and model intelligence’. They acknowledge that every compute-hour spent on capabilities raises the stakes for alignment, which in turn piles on the pressure as more capable models appear on the horizon.
The concern is that alignment and interpretability researchers are squeezed. Breakthroughs in capabilities (and market demands) can’t wait, even though understanding or controlling the models may be slower.
Anthropic pitches interpretability as an ‘MRI for AI’ that must arrive in time to check progress because AI is ‘opaque in a way unprecedented in the history of technology.’ This is no doubt true, though it is a function of the fact that (A) symbolic approaches where you can see logical operations turned out to be rather brittle, and (B) the connectionist models that supplemented them get harder to understand as they grow in size.
Labs like Anthropic are now investing in a handful of AI safety startups. Basically, they are treating interpretability as a competitive advantage. This is a good thing. Developers have a vested interest in keeping users safe after all, and people generally want to work somewhere that takes minimising harm seriously. Of course, the sceptic will say that the labs are just doing this for the headlines. And while I think there’s some truth on that count, if I had to guess, I would expect that is not the primary motivation behind safety work.
But this strategy contains an interesting tension. Labs openly admit today’s systems are largely black boxes, yet nonetheless deployment continues (after a round of evaluations and a bit of user feedback). OpenAI’s ‘iterative deployment’ strategy, for example, involves releasing ever-more-powerful systems to learn from how people use them in the real world. Clearly there is a limit to what can be learned in the laboratory–and it is important to introduce powerful systems gradually to allow people and institutions to adapt–but I struggle to find an analogous example of a similar technology deployed in such a way.
The upshot is that the bus is on the road, but its steering is still being worked out. AI firms feel they need to create ever-more capable AI to avoid being left behind by the competition domestically and internationally, while at the same time seeking to understand those systems for safety and policy reasons. This drive is a defining feature of the current moment, as labs must satisfy investors and regulators that AGI is both inevitable and controllable.
We’ll know it when we see it
Not long ago, the idea of general-purpose machine intelligence was confined to the backrooms of philosophy departments, LessWrong, and some of the more adventurous long-range strategy groups. Today it’s a line item in investment memos and policy briefings. Labs invoke it to define their missions and regulators write it into their frameworks.
The closer we get to AGI, the less clear we are about what it really is. Definitions remain contested, timelines volatile, and safety strategies unproven. And yet the momentum is undeniable. More money leads to more capability, which leads to more confidence, which leads to more money.
That’s why I think the most honest description of the current state of play is neither utopian nor apocalyptic. It’s ambivalent. The people building these systems believe something big is coming. They are working hard to understand it, but not at the expense of deploying it.
Like Potter Stewart and his definition of obscenity, we may never fully agree on what AGI is. But the builders and their backers reckon they’ll know it when they see it. And they’re betting the house that they’ll see it soon.
Editor’s List
Articles on AGI:
Thank you for reading.
writes Learning from Examples: A history newsletter about the future.
I have a community chat going on AGI here, that might be a better place to have production dialogues: https://open.substack.com/chat/posts/9ce993f6-313f-4a0b-92c6-21b07d46e21f
Cheers! Yes. I am too certainly behind... I suppose it's a question of whether incremental rather than fundamental changes are needed to create something AGI-shaped... Are current techniques the Blu-Ray to neural net's DVD, or are they the birth of Netflix?